Assessing the Efficiency of AI-Driven Development: 2 methods of quantitative evaluation of the impact of AI usage in Software Development
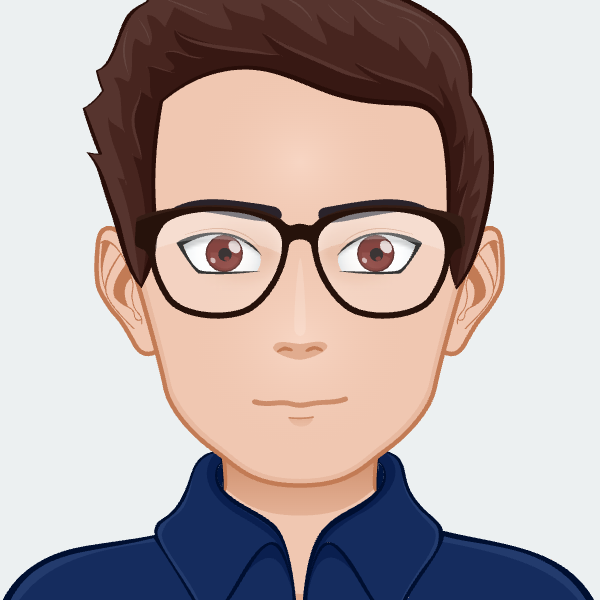
The rise of AI-powered tools is transforming software development, promising increased efficiency and improved code quality. But how can organizations objectively measure the impact of AI on their development teams? This article outlines a structured approach to evaluate the actual impact of AI assistance in Software Development.
We will look into two different approaches to conduct this quantitative evaluation. The first approach consists in evaluating the impact of AI tools uniformly in a given team, by comparing the overall Software Development Life Cycle (SDLC) performance before and after implementation of AI tools, whilst the second is comparative, and will compare the SDLC performance between 2 groups of developers: one that uses AI tools, and the other one that does not.
The first approach allows for a faster deployment of AI tools in the development team, as all members of the team are setup with the AI tools used to assist the Software Development. It is therefore allowing for the tools to be deployed more rapidly.
 With the second approach, the AI tools deployment will span across a longer period as by principle it is necessary to wait for the end of the assessment period to evaluate the benefits of using the AI tools for software development before deploying largely to all team members – assuming the results are compelling enough to do so.
 As a result, the second approach mitigates the investment financial risk better than the first one. Indeed – should the deployment of the AI tools prove not beneficial or not sufficiently compelling from a Return of Investment (ROI) point of view, the budget increase will be limited to the group testing the AI tool. With the first approach, the cost of the AI tools is greater since all developers have been setup.
The first approach is also more sensitive to the impact of external factors than the second approach – which could skew the results of the assessment. External factors such as changes in project requirements, team composition, company’s strategy, or market conditions for instance will indeed by definition affect the entire development department. Therefore with the first approach, it becomes difficult to evaluate the before/after implementation of the AI tools for their own merits – given the fact that everyone has been impacted by the external factors – resulting in the question: are the changes in performance due to the AI tools or to the impact of the external factors?
The second approach allows to mitigate external factors better: everyone is still impacted by them, but because a group is using the AI tools and the other one isn’t, it is possible to compare the actual impact of the AI tools despite the impact of the External factors because we are presented with 2 different configurations for the same baseline.
Evaluating the effectiveness of AI-driven development requires a multi-faceted approach, examining its impact on both team performance and individual developer productivity. Here’s a breakdown of key areas to focus on – with a before implementation of AI tools VS after implementation of AI tools, leveraging development analytics insights :
DORA (DevOps Research and Assessment) metrics provide a robust framework for evaluating the overall performance of a development team. By comparing these metrics before and after AI implementation, you can gain a clear understanding of its impact on key areas:
Analyzing the Issue Cycle Time provides a granular view of the development process from backlog to release. By tracking the time spent in each stage – pickup, implementation, QA, and release – you can identify bottlenecks and measure the impact of AI:
Zooming in further, analyzing pull request cycle time offers adetailed view of the coding process itself:
Measuring individual developer productivity is crucial for understanding the impact of AI at a granular level:
AI can significantly influence where engineering effort is allocated:
In this section, we are looking at a structured approach to comparing the efficiency of two development teams: one embracing AI-driven development and another using traditional methods. The underlying principle of this assessment is similar to the efficiency test of a new medicine, where one group is given the medicine to test, whilst the other one (control group) is given a placebo. In our case, it’s of course not about giving any “placebo” tool to the control group, but rather insuring that it sticks with traditional methods of Software Development, whilst the test group uses AI tools to assist their software development.
Before initiating the comparison, establish clear objectives and identify the key metrics that will be used to measure the impact of AI. Focus on quantifiable aspects of the software development lifecycle (SDLC) where AI is expected to have a significant influence. Consider the following:
Choose two teams with similar skill sets, experience levels, and team sizes. They should ideally be working on projects of comparable complexity and scope. This ensures that observed differences are attributable to the use of AI rather than variations in team capabilities or project characteristics.
Although this is fundamentally a complex metric, dependent on human perception, it is possible to get a tangible evaluation of the similarities and differences in terms of proficiency of developers with the Engineering Proficiency metric, that provides insight into an individual engineer’s experience and expertise. From there, it is easier to identify commonalties and ensure the teams used in the assessment are of similar skillsets and experience, working on projects of comparable complexity and scope.
Before the AI-powered team begins using AI tools, collect baseline data for both teams across all defined metrics. This provides a pre-AI performance benchmark for comparison. Collect data for a sufficient period to account for natural variations in team performance.
Introduce AI tools to the designated team incrementally, starting with areas where they are expected to offer the most immediate benefit, such as code completion, automated testing, or vulnerability detection. Provide thorough training and support to ensure developers effectively utilize the new tools.
You can verify the level of acceptation of the new tools. For instance, GitHub Copilot now offers to quantify the levels of utilization.
The assessment will only be valid if the team using AI tools actively uses the tools in question of course.
Regularly collect data on the defined metrics for both teams throughout the evaluation period. Employing automated data collection and analysis tools, such as Keypup, can significantly streamline this process. Analyze the data frequently, looking for statistically significant differences:
While quantitative data is crucial, qualitative feedback offers valuable context and insights. Conduct regular surveys and interviews with developers on both teams to understand their perspectives:
Be mindful of external factors that might influence the results, such as changes in project requirements, team composition, or market conditions. Document these factors and consider their potential impact on the analysis.
Treat the evaluation as an iterative process. Use the gathered insights to refine the use of AI tools, adjust development workflows, and address any challenges faced by theAI-powered team.
And of course, measure again the same objectives and metrics to quantify the evolutions.
Example: Analyzing Defect Density
If the defect density in theAI-powered team decreases significantly after the introduction of AI-assisted code review and testing tools, while the control group's defect density remains relatively stable, it suggests a positive correlation between AI usage and improved code quality.
‍
By implementing a structured comparison between AI-powered and traditional development teams, organizations can gain a data-driven understanding of AI's impact on software development. This enables informed decisions about AI adoption, optimized development processes, and ultimately, the delivery of higher-quality software more efficiently. The key is to maintain a continuous feedback loop and iterative approach, adapting strategies based on observed data and developer feedback to fully realize the potential of AI in software development.